Crack Segmentation for High-Speed Imaging: Detection of Fractures in Thermally Toughened Glass
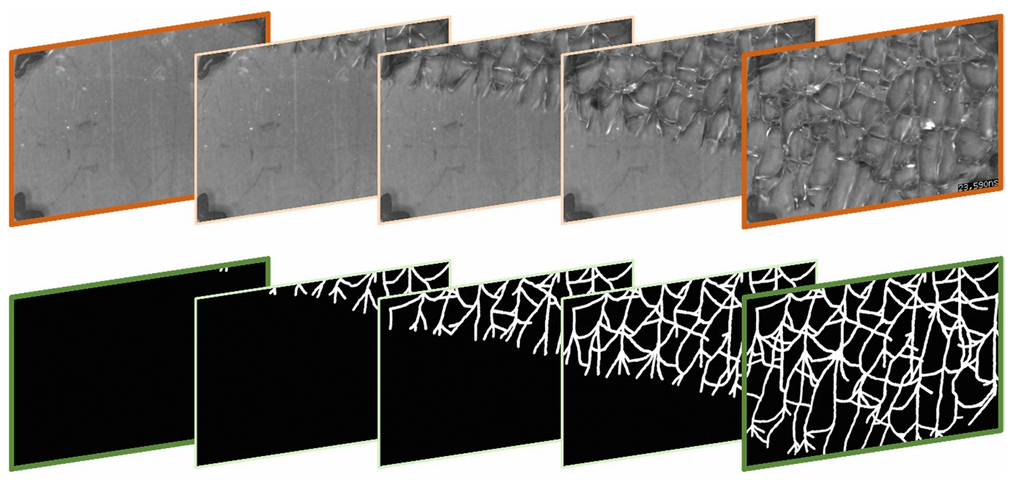
Abstract
Fracture morphology characterization in broken glass panes is crucial for designing laminated safety glass (LSG) in civil engineering. Verifying completely broken LSG systems requires destructive sampling, increasing costs and hindering development. Therefore, to determine the residual load-bearing capacity, the link between the pre-fracture characteristics and the fracture morphology must be
known. However, when the crack propagation needs to be directly captured with high-speed imaging, conventional methods are no longer sufficient for detecting cracks. To enable such investigations, we propose a novel machine learning framework for crack segmentation in high-speed imaging that addresses the complexity of glass fracture and minimises the required labour costs. In this study, the crack propagation of a sample was recorded and analysed at 2,000,000 images per second. The results showcase accuracies surpassing 97% while requiring only two labeled images for training, thus streamlining practical implementation. Furthermore, we show the method's robustness to the extent that hyperparameter tuning becomes unnecessary. Instead, we offer guidelines for selecting the most crucial hyperparameters depending on the problem. Our method offers a promising approach for non-
linear temporal interpolation of noisy images, with implications for various applications extending beyond glass fracture analysis.
Published
Issue
Section
Experimental & Numerical Investigations
License
Copyright (c) 2024 Henrik Riedel, Leon Bohmann, Frank Bagusat, Martin Sauer, Miriam Schuster, Matthias Seel

This work is licensed under a Creative Commons Attribution 4.0 International License.